Finance
Chaos and Complexity Economics (with J. Doyne Farmer)
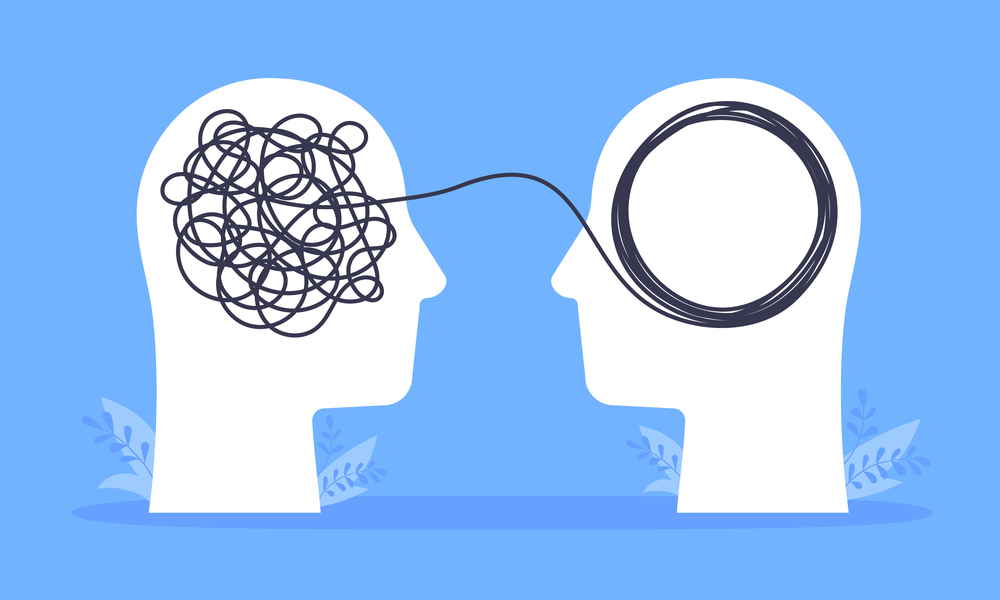
Intro. [Recording date: August 1, 2024.]
Russ Roberts: Today is August 1st, 2024 and my guest is physicist Doyne Farmer. He is the Baillie Gifford Professor of Complex System Science at the Smith School of Enterprise and the Environment at Oxford University, where he’s also director of the Complexity Economics Program at the Institute for New Economic Thinking at the Oxford Martin School. In addition, he’s an external professor at the Santa Fe Institute.
He is the author of Making Sense of Chaos: A Better Economics for a Better World, which is our topic for today. Doyne, welcome to EconTalk.
J. Doyne Farmer: Thank you. Happy to be here.
Russ Roberts: Let’s start off with the fundamental idea behind your book and much of your career, which is the idea of complexity economics. What does that mean to you? What is complexity economics?
J. Doyne Farmer: Well, simply put, it’s the applications of complex systems, science, and methods, to economics. And more specifically, that means doing economics in a different way than mainstream economists do it. It means simulating the economy rather than using utility maximization to write down equations to solve for what people will do.
Russ Roberts: So, I’m trained as a standard economist, more or less. Sometimes more, sometimes less. And, I’m sympathetic to a lot of your critiques of economic theory, but in other areas, I’m going to try to defend them.
Certainly you’re right that, in the standard economic model, people are motivated by some form of maximization, typically utility being–a relatively empty phrase–meaning whatever they happen to like. And people try to get as much utility constrained by the fact that they have finite income. So, that’s the economist’s model.
The only really important thing that comes out of that model–and you may disagree. But, in my mind there’s only two things are important that come out of it. One is people buy less of something when the price goes up and more when it goes down. They respond to price incentives.
And it also allows economists to talk about welfare–wellbeing–and that’s very important. I think it’ll come up a little bit in our conversation today.
That’s a pretty modest claim about human behavior that economists are making. At least the first one: people respond to prices. What’s different in complexity economics in how people are behaving?
J. Doyne Farmer: Well, I think the key difference is, first of all, I’m interested in making quantitative models. Models that make predictions that have numbers attached that you can believe and that have enough richness and detail in them about institutions that if you’re thinking about a policy question and you want ask what-if questions, you can get answers that you can trust.
So, I agree with you that those features you just mentioned are nice, but you mentioned them in a very qualitative way, and I don’t think that takes us far enough.
I also think that when you start building models that really have enough in them to give you reliable answers, that the problem with that formalism you mentioned is that in order to derive with equations what the optimal decision for people is, subject to their beliefs, you really get stuck as soon as things start to get complicated. Complicated enough, I would argue, to put in all the essential features of real-world problems like climate change or macroeconomics more generally. So, I think that’s the side of this that I’d like to stress.
Russ Roberts: There’s a certain inconsistency, obviously, in mainstream economics between the models that are written down, the formal models, theoretical models of, say, equilibrium or individual decision-making over time. Any of these. They’re not quantitative: they’re mathematical. Those are not the same thing. But, economists typically build models that are mathematical. Then they’re stuck with the real world where they’ve got all this data and they’re very happy. Economists are very happy to proceed with those numbers as best they can. And, a lot of what you’re critiquing, I think about economists’ vision of, say, individual behavior or even market behavior is just shunted to the side and economists just look at the numbers and they’re okay with that.
Do you think the complexity economic framework gives you a different way of thinking–I know you do, so tell me what you think is different about that framework for prediction. Whether it’s in response to a macroeconomic event–a rise in interest rates, or COVID which you write about hitting the economy, or climate change, or the financial crisis of 2008. These are all things where–you know, when economists, they often did a very bad job of predicting ex ante what was going to happen. And ex post, they have the data so they fit them in certain ways and tried to explain certain things and maybe even postulate what could have been done about it at the time; but it’s very imperfect. And, why do you think that your approach would be better?
J. Doyne Farmer: Yeah. So, let me just emphasize first that my book is really not a critique of mainstream economics. I actually removed all the stuff criticizing. I don’t want to make mainstream economists angry. And, even if I do have some criticisms, I just let them lie because I really want to focus on the alternative.
And, if we are going to critique the mainstream, my main critique would just be: Allow more room to let these other ideas in and let them compete against your ideas. Let’s let data and empirical facts carry the day to see who is right in what circumstances. Because I also think there are cases where I think the complexity economics way of doing things has clear advantages, and there’s other cases where the mainstream way has advantages.
So, I think specifically complexity economics has advantages when things get complicated–when you have a messy situation and where you are worried that many different factors matter at the same time. And, that’s when I think the complexity economics approach can do better.
Of course, at the end of the day, what matters is ex-ante prediction. The predictions you make before things happen, those are the predictions you’re going to make that people are going to respond to and that count.
Of course, you always want to go through and do a postmortem to figure out what you got right and what you got wrong and why did you get wrong what you got wrong; and try and change your models in hopes that you’ll do better next time. And, economics is a really hard topic, so I don’t want to throw stones at scientists for getting it wrong. It’s really hard. As a physicist, atoms don’t think. They’re ultimately much simpler and easier to understand than people. But, on the other hand, we really want to get this right because economics matters. It makes a big difference in people’s lives. It makes a big difference in world events; and economic policies that are wrong can have very nasty side effects.
Russ Roberts: Yeah. I agree with all that. Let me ask it–try to get at some differences and similarities from a different direction.
I think some economists make the mistake of building a model of human behavior, getting an implication from that model, find it confirmed in the data, and then concluding that the model is accurate–meaning an accurate portrait of how people behave. I think that’s wrong, and I think you probably agree with me.
But I would also add that in economics–at least the kind that I’m talking about–we don’t just care about prediction. We also want to understand. We want to have an understanding of either the human being and the way choices get made by human beings. We might want to understand emergence that you’re as interested in as I am and how things emerge out of individual decisions from the bottom up. And, it seems to me that economists are not very good at that–at the first thing–at trying to really understand how people really behave. And, behavioral economics was a response to that. The question is–and complexity economics is another response to that. The question is: What do we gain from that? It’s true, we can in theory get a better understanding of individuals. But I think you are claiming we also get better predictions. Is that accurate? Am I right?
J. Doyne Farmer: That’s right. That’s right. But, let’s sort of parse that into a couple of different pieces.
There are certainly situations where having better models of human behavior going into the model that’s then used to make economic predictions–that’s where that’s going to work better. And, I think economists will broadly agree with that. As you said, there’s a big field now of behavioral economics. But, I would argue that there’s cognitive dissonance between the behavioral economists–who are saying this is the way people behave in economic settings–and the macro-modelers who build models for places like the Federal Reserve or the U.S. Treasury–who are saying, ‘Here’s our model for what’s going to happen if we follow this policy or if these events happen.’ And, those two things are at odds because right now the models for places like the Federal Reserve are still based essentially on rational expectations. They add on what they call frictions, which constrains rational expectations. And, they’ll acknowledge: This is not a realistic model of how people actually behave, but we don’t know how to bring real behavior into our models because it’s too complicated and we can’t write down the mathematical equations to put it in there.
Now there’s some proposals for doing that, but none of them have really gained traction. It’s not clear that any of them work better than the standard rational-expectations-based models. So, my view is economics is at a crossroads where it’s confused. Where, any sensible economist has acknowledged that people are not rational, but they don’t really know yet how to put that into the models–the workhorse models, as I call them–that we use to make policy.
So, I see complexity economics as a way to resolve that, because we’re not limited by complication. If people behave in a more complicated way, fine. We can write a computer program that mimics that. And, we don’t have to solve equations to get the answers. We put them into our computer simulations and they deal with them just fine. And, maybe most importantly of all: Models are tractable. I quote several mainstream economists in the book saying, ‘Once you have more than, say, a dozen independent variables in a standard model, you can’t solve it anymore. The solution times are measured in centuries.’ In contrast, we have models with a million agents in them all acting of their own volition. And, we can do that because putting in decision rules for how people make decisions more realistically actually makes it much simpler to run the models; and complication doesn’t bog us down in the way it does in a mainstream model.
Russ Roberts: Let’s look at something a little more specific so that listeners can get an idea of what the distinctions are.
Let’s talk about the housing market. We know–those of us who’ve lived some length of time, enough to be an economist–people know from real life experience that sometimes people will, say, get angry at a potential buyer who is disrespectful either to seller talking about the seller’s house and the seller will sometimes say, ‘I’m not going to sell to that guy. I don’t care if he’s the highest bidder. I’m not going to sell to him.’ Now, I’m not interested in–I don’t want to debate whether that’s rational or irrational. We could take interesting perspectives either way. But we understand that oftentimes narrow monetary self-interest doesn’t explain everything. Okay. So, that’s a good insight. It’s true.
But if I want to predict, say, how the housing market of a particular city is going to respond to some kind of change, I don’t think I have to deal with the fact that some people have strange preferences, strange whatever. I just aggregate; I don’t worry about it. I just assume there’s a demand for housing. Not my field–area–when I say “I.” Economists would just assume that.
And, what you’re trying to do–and this is, I want to let you give listeners an insight into this–what you’re trying to do is you want to take a million people and let them behave in all kinds of different ways the way human beings actually behave. And so, what you’re interested in is called Agent-Based modeling, meaning you model the individual agent and then you’re going to allow all kinds of rules of thumb, heuristics, and other things that human beings probably do–they’re not actually sitting around making a utility-maximizing calculation in their head.
What I want you to try to explain is why that Agent-Based approach is likely to be more successful in your view than the aggregate-demand-for-housing way, which just says, ‘I don’t know why people do what they do. I don’t care. There’s just a demand for housing in Washington, D.C., or Seattle, Washington, or Topeka, Kansas. And, if I change, say, the capital gains tax or I change interest rates, I have a simple model that predicts what’s going to happen and it’ll do fine.’ Why do I need to go to the level that you’re doing? What do we gain? And, try to give people a feel for that.
J. Doyne Farmer: So, you would gain several things. First of all, I don’t think the example you gave at the beginning of somebody getting angry is–I mean, I wouldn’t know–in our models, we wouldn’t be able to understand who the angry people were and how they would get angry. So, that’s not the kind of thing that we’re trying to do.
But the big difference comes with things like: How do you set housing prices? Right? In all standard economics models, housing prices are set through market clearing–meaning you equate supply and demand. You can write that down mathematically. You can solve the equations.
But, how do housing prices really get set? They get set by what’s called ‘Aspiration-Level Adaptation.’ That is, the seller when they’re selling their house, goes to the real estate agent. The real estate agent helps them find some comparables. They between them decide on a price that they think is more or less the appropriate price they could hope for if everything goes well. They put that house on the market. If it doesn’t sell after a month or two, they mark it down. If it still doesn’t sell, they market down again. They keep marking it down until either the seller says, ‘This price is too low. I don’t want to go lower than this. I’m just not going to do it.’, or the house sells.
And, I can say that’s how they do it because we looked at millions of sales in Washington, D.C., because we had access to a decade-and-a-half worth of housing data where we could see every price a house was offered at and whether or not it sold and what price it sold at.
Now, that might sound like a small thing, but it actually makes a big difference. Because it means that prices react very sluggishly to changes in the housing market.
It also means that the market can be far from clearing. You can have 20 times as many buyers as sellers, or vice versa. And, during something like the housing bubble that popped in 2008, leading up to the bubble, you had far more buyers and sellers. And then when the bubble popped, you had far more sellers than buyers. And that makes a big difference in the way the prices actually moved.
But, the second thing, if you look at our model–maybe the second and third thing–a second thing is that we could really look at the details. Because: what caused the housing bubble? The housing bubble was caused by a shift in lending policy by banks. Banks got a lot looser in who they were giving loans to. And, in our model, we actually looked through all the loans that were given in Washington, D.C. area. We looked at the criteria of the buyers behind those houses. And we could just see the way the lending policy shift by seeing what the characteristics of those loans were. And, so, it was a combination of several things: that you went from the old-fashioned vanilla 30 year loan, fixed interest rate, like the one I had on the first house I bought–
Russ Roberts: 20% down–
J. Doyne Farmer: 20% down. Oh, sorry, yeah, 20% down. Fixed interest rate like the one I had, to much more complicated loans with balloon payments, smaller amounts down.
And, so, because we were doing a simulation and not a mathematical model, we could put all that detail in. We could, you know, put the kinds of loans that were actually given and see how changing lending policy in all of its detail affected the bubble.
And, part of what we saw in our simulations was that that was really the dominant effect. That’s what fueled the bubble. We could compare it to, say, interest rates, which had a little bit to do with the bubble. But we could see that these much more complicated loan types, which were much looser, was a thing that fueled the bubble.
Then, the final advantage of the way we did it is–that has not been fully exploited in complexity economics yet–is that we had both a micro-model and a macro-model. That is: We were really simulating the behavior of individual house sales. And there is the capability to really match that up one-to-one with the world to advise: ‘Well, on this block things are different than they are on this other neighborhood over here,’ or ‘These kind of buyers are affected differently than those kind of buyers,’ or ‘These kind of sellers and those kind of sellers.’
So, we had rich textural detail in our model that you just can’t get in a mainstream model.
So, those were the really three biggest factors I think that made the difference and really allowed our model to be much more realistic and accurate and useful than the mainstream models.
Russ Roberts: Yeah. It’s interesting because any good economist would tell you that those things all would matter. Right? You don’t have to be a behavioral economist or a complexity economist to understand that.
But there are often–the statistical models of the, say, housing market often abstract from that level of detail. And so, like you say, they literally have nothing to say about that. Certainly ex-ante. Ex-post, they say, ‘Oh yeah, we should have had a variable for that. We didn’t know.’
And, I think that’s a fair criticism.
And I would even go further and say that–you point out in the book at one point that economists weren’t very worried about drop in housing prices. I think that was also in advance of 2008, I think they also totally misunderstood, including myself, how housing prices and the financial system interacted with the macro economy. I think it was a terrible blind spot. Just didn’t know anything about it. And, I think that–you go ahead.
J. Doyne Farmer: Let me actually correct that. Because. it’s not that they weren’t worried about it. They were worried about it. The economists at the Fed who I know were quite worried about it.
And, the problem was they asked FRB/US, a Federal Reserve Bank US model, their best model, what happens if housing prices drop by 20%? And then, said, ‘Oh, not much. No big deal.’ And, as they themselves said, with hindsight, the model was off by a factor of 20. So, they were worried. They were good economists. It was their model that let them down.
Russ Roberts: And, their trust in that model or their willingness to lean on it.
Russ Roberts: So, I think that’s a fair criticism. I think the real question is whether–not after the fact, but ex ante. The challenge is, ex ante, it’s always hard to know what belongs in the model.
Now, if you’re doing what you’re doing, looking back at past data, it allows you the potential to uncover factors you might otherwise miss if you’re thinking about it at the individual level. And, I think that’s a good criticism of the standard ways that macro economists and others model these–what are fundamentally general equilibrium problems, right? A thousand things interacting at once. And since in real life they don’t do it smoothly, things often will turn out in ways that are not so easily coped with.
I want to stop–I want to go back to that for a second about the stickiness of prices, which you alluded to. If people make decisions the way you suggest, which I think they do in the housing market–that is not totally inconsistent with the standard mainstream way of thinking about prices as the result of market forces.
What–those of us who believe in that model are not very good at change. Or at least I would say the period between A and B where the change takes place. We don’t have any understanding of that process.
And, I think one of the other things that you’re capturing in an agent-based model is trying to get at that. The frictions that economists often will just ignore and say, ‘Well, I don’t know how we’re going to get there, but eventually the market will settle down at a higher price–or a lower price, or whatever it turns out to be,’ with no understanding of how we get there from here. And I think that’s a potentially valuable thing we can learn from your kind of–the kind of simulations you’re talking about. Do you think that’s right?
J. Doyne Farmer: Yeah. Because the way we model the world is intrinsically dynamic. If an equilibrium happens, it’s an emergent phenomenon. It’s something that the model does. And model comes back and says, ‘I think things are going to settle into an equilibrium.’ But, the model doesn’t necessarily say that. It very often–because we’re modeling in a disequilibrium way and we’re actually explicitly making a dynamical model, we have the capacity to get the dynamics right, to get the path from A to B rather than just saying, eventually we’re going to land on B.
Russ Roberts: Are there any constraints imposed on–on that path? I understand that any one person can be way out of whack. I had a neighbor who said what he was going to offer his house for, and I thought to myself–I said to him, actually, ‘I think it seems a little high.’ He said, ‘Yeah, but I only need one buyer.’ I’m thinking to myself later–I didn’t want to say it in his face. That’s true. But it’s going to be really hard to find a buyer if you’re way out of line with the comparable, say, which you alluded to earlier.
So, you want to have some constraint on the whole system of supply and demand, it would seem like, and not just rely on individual motivations and so on. Because people do learn. They’re not rational in making perfect predictions, but they do learn from the constraints of the system. In my view. Do you agree?
J. Doyne Farmer: I totally agree.
And of course, we can put and often do put learning into our models and let people adjust. We’re interested in how the herd behaves typically rather than how a few isolated individuals behave. Although I think one of the big strengths of our models is that we can deal with heterogeneity. We can deal with a world where people are different.
And, which becomes important say in macroeconomics where poor people behave very differently than wealthy people, and we can really accurately get at that.
That is one of the hottest topics in macroeconomics these days–in mainstream macro-economics.
I would argue that we can do it better because when they try and put that in, they have to put it in in a very stylized way. They have a distribution of infinitesimal people and they can only really get at one feature.
In our models, we can put in income, race, gender, geography. Whatever you want, we can put it in there. And we can–we in fact do build synthetic populations in our macro models. We might have a million individuals in our population. Those individuals are chosen using census data to match real people.
And then, we can get at things like the fact that, like, consumption behavior really depends on how old you are, how much money you have, and maybe even depend on things like where you live or what your education level is.
Russ Roberts: Shouldn’t all those things be in the mainstream models as well the only difference being that you’re doing a simulation? We understand saving rates differ by age, we understand they differ by income, we understand they might differ by education. And, if you have the data, you would go out and try to measure those. Just separate impacts of each of those variables. If you’re doing a simulation, you can include it, but what’s the significance of that if you don’t have the actual data from the people and the real situation you’re looking at?
J. Doyne Farmer: Let’s make a careful distinction between two kinds of economic models. One is a statistical model often called econometrics in economics where you just take the data, you typically fit linear function to the data and you make some references about what people did. And, you could then use that model to make predictions about what they’ll do in the future. As opposed to first-principles model, that we mentioned before where you write down utility functions for the agents, you write down the equations, you solve those equations, you say, these are the decisions people will make, and these are the economic consequences of those decisions.
And so, we’re really talking about the latter here. Because we are providing an alternative for that.
And, the problem is, in making that kind of model, you can’t deal. It’s just intractable. It’s unfeasible to in age and income and everything else. Right? The state-of-the-art models deal with income. Period. You just can’t put in all these complicated things and solve the equations.
And, that’s where we have a big edge.
Russ Roberts: But, that comes back to my earlier question–give you a chance to maybe clarify it here. Those people writing down those equations in mainstream economics, you’re a hundred percent right. They can’t cope with the complexity that you’re able to cope with.
But, when they come down to the econometrics, they don’t worry about it. They just look at the data. So, why is it important that an econometrician, at least in theory, has some kind of utility-maximizing model in the background?
In fact, most–a lot of–economists that I talk to on this program and out in real life say, ‘Yeah, theory is a waste of time. I just see what the numbers tell me.’
Now, I think that’s an impractical attitude for a variety of reasons. You can talk about it if you want. But I think so many of econometricians use those mathematical models that you’re right are highly limited and then they just wave their hands when they actually get to the data. That’s my experience.
J. Doyne Farmer: So, first of all, I’m not challenging econometrics. Econometricians are doing some good stuff these days and I’m not even offering an alternative to that. I’m offering an alternative to the more theoretical models.
And, the reason you need the more theoretical models that have causal relationships built into them is when you want to consider counterfactual situations. If you want to say, ‘What if we make a policy change?’ If you’re an econometrician, the only way you can understand what happens under a policy change is if you have historical examples where people did natural experiments and made those policy changes. If you do, you can go see what happened and you may or may not get reliable answers because often maybe you only have two or three examples where those policy changes got made so you got to worry about statistics.
But, if you want to consider counterfactual situations, you really have to understand cause/effect because, when you make a policy change, you’re going into a world that may be different than any world you’ve ever seen before. And, that’s where these kind of models become essential. And, the econometrician will wisely say, ‘Sorry. I can’t say anything here.’ And, that’s really what complexity economics is about, is providing alternative to causal models that have causal relationships built in and that try and make predictions from some version of first principles.
Russ Roberts: You make a number of claims in the book about the effectiveness of the models you’re using–and seriously interested readers can go back and look at the original work and assess those and make their own judgment. But what’s clear is that the approach you’re talking about, which you’ve been doing for a long time–and my hat’s off to you for that–have not managed to infiltrate the mainstream economics profession. Why do you think that is?
J. Doyne Farmer: It’s a mixture of two things. One is that complexity economics is a new field with a small number of participants, so it’s really a David and Goliath, but David is a hundred times smaller than Goliath here or more. It’s that right now–so let me just say we don’t have that many models–we don’t have a model for everything yet. And so, the competition is just forming up. The race is really beginning, in a way.
Now, that said, I give some examples in the book like our model of COVID where we produced a model that made ex-ante predictions ahead of the fact, real time, and we totally nailed the answer. We predicted a 21.5% decline in GDP [Gross Domestic Product] in the United Kingdom in second quarter of 2020. The answer was 22.1%. And, we predicted lots of other things in detail that more or less were right.
And, the model has since been run in other places, not ahead of the fact, but the same basic model. It did well in other countries, too.
So, we now have some proofs of principle that these models can do well. We also have examples of macromodels that seem to be doing about as well as mainstream macromodels in the places they’ve been tested. That’s already impressive because these are models built by a couple of people, and have[?] very little history behind them. In contrast, mainstream models have many, many decades with hundreds of people working on them in every decade, and so the effort is much, much bigger. So, we’re really beginning to just see that difference playing out.
And, my prediction is that what we’ll see over the next decade as these things begin to be scaled up and as we really begin to have a head-to-head race where we can see who is doing better, we’re going to see more and more examples where the complexity economics models do well.
My other affiliation that you didn’t mention is as chief scientist and director of a company called Macrocosm that we’ve recently founded. The goal of that company is to scale up these techniques and reduce them to practice so that if I get called by reporters saying, ‘What do you think is going to happen to the economy in Nigeria next year?’, I can give them an answer. And so, we really start to accumulate a large track record of real predictions and we run a proper head-to-head race.
Russ Roberts: I think you were going to say something else about–anthropologically–about the challenge of–
J. Doyne Farmer: I was. Thank you for reminding me. I have to try and find the right way to say this–
Russ Roberts: I understand.
J. Doyne Farmer: But, economics is a fairly closed profession. If you’re a graduate student in a mainstream economics department and you go to your advisor and say, ‘I’d really like to build one of these agent-based models because I think it looks really cool.’ And, your advisor will say, ‘Sorry. That is really a very bad career choice. Because if you do that, you’re never going to get a job at Harvard or any other American university in an economics department.’
And, that’s just the truth. Because, somehow the field has gotten locked in to a certain point of view and they’re very skeptical and not willing to really let in other points of view yet. That’s part of why my theory of change, here, is: Let’s start with commercial applications. Let’s also focus on Central Banks because actually several Central Banks are open to these kind of ideas and are running models that are very much like the Washington housing model I mentioned. Bank of Canada is starting to run an agent-based macro-model. Bank of Italy. Several Central Banks are starting to use these ideas because their reputation is on the line when they make bad predictions; and there’s less dogmatism; and so they’re more open-minded. And so, I think once we start to really infiltrate those channels, then that will start to put pressure on economics departments to admit complexity economics under the tent.
Russ Roberts: And, I think that could happen.
I was going to suggest another reason which, I’d be curious of your reaction to it. Some of it’s marketing. So, when I teach supply and demand to a freshman economics student, it’s very cool. It may not be accurate. I concede it’s not a precise description of how prices actually get determined. It’s a shorthand way of capturing the reality that you can’t just set price at whatever you want when you’re selling a house, and you can’t just decide you want to pay a certain amount for a certain quality house when you’re buying a house. And, it’s a primitive way of organizing your thinking around that question.
I think complexity economics to some extent suffers from the fact that it’s not as easy to describe about what’s different. It’s clear that it’s an attempt to make a, quote, “more realistic” model–a richer model of human decision-making. But, it doesn’t have the, I think, some of the elegance that mainstream economics has.
And, I would say that the complexity economics that is in main mainstream economics, which would be Adam Smith and Hayek, both of whom cared a lot about emergent phenomenon and whether they called it that or not–that doesn’t fit very well in the models either. And, it’s off to the side. Like supply-and-demand: that’s emergent; and it is, but it’s thin.
And so, I think the richness which is part of the Austrian School of Economics, is also on the outside looking in because it doesn’t have the simplicity and elegance of mainstream economics that’s been developing over the last 75 years or so, beginning with Paul Samuelson and The Foundations of Economic Analysis. So, the elegance isn’t there. And, as a result, it’s harder to compete, I think. And, maybe that’ll change. Do you think that’s true?
J. Doyne Farmer: No; I think you’re right. People like things that are elegant. I’m a physicist: I like math. I’m have nothing against math. I use it all the time.
And, even in agent-based modeling, we use math, too. There are several examples: in the book, I mention–I don’t really go into–using theoretical approaches to understand what’s going on at a more conceptual level. Those theoretical approaches are drawing on other fields–on ecology, on statistical physics, and other domains–because once you get away from utility-maximizing agents, it becomes natural to pull other ideas in to get more qualitative understanding about what’s really going on. And, qualitative understanding is a really good thing.
I just want to say one other thing in response to what you said. I’m not against supply and demand. Supply and demand is a big force. And, in fact, all the models that I discuss in the book have supply and demand in them in one way or another. Some of them even have market clearing, which means supply equals demand.
But, when supply is greater than demand, then we know that prices are likely to go down; and when demand is greater than supply, we know they’re likely to go up. And, I also discuss these kind of dynamic supply and demand models. But, supply and demand is, it’s one of the things in economics you can really grab onto because it works. There’s no doubt that supply and demand is a really major force that is an underpinning of economics. And, there we agree with a mainstream economist.
I think our edge there is that we can really talk about out-of-equilibrium situations where supply doesn’t match demand and what happens on the way to supply equilibrating with demand, which it usually eventually does.
Russ Roberts: I think that’s a very good summary of what’s especially distinctive about what you’re trying to do. We touched on it earlier.
Russ Roberts: Before we continue, I want to mention one thing that comes up in the book that was kind of fun for me, which is: in your youth, you had an adventure at the roulette table. It happened to be something I’d read about in another book called The Eudaemonic Pie, by Thomas Bass. Give readers just the shortest thumbnail of what you did in that time and how it affected your way of thinking about these kind of issues we’re talking about, because it’s quite extraordinary.
J. Doyne Farmer: Well, thank you. So, the brief summary would be, when I was a graduate student of physics, my friend Norman Packard and I–and actually a group of about ultimately 20 other people–beat roulette. We did it by predicting where the roulette ball would go after the croupier has released it.
That is, we built the first wearable digital computer, which was concealed under an armpit with a pack of 12 AA batteries under the other armpit. And we had switches in our shoes. When the rotor–the central piece of the roulette wheel–when the zero passed a given reference point, we would make a click, we would click again, then we would start clicking on the ball after the croupier released it. The computer would make a prediction about roughly where the ball was likely to land, about 6-10 seconds ahead of when the ball actually landed. And then, we would send a signal to another person who would lay bets down on numbers that were in that part of the wheel.
So, this was a fantastic adventure. We called our company Eudaemonic Enterprises: hence The Eudaemonic Pie. And, so, it was a lot of fun.
But, it taught me some remarkable lessons. For one thing, it taught me a lot about how you make models that actually make good predictions.
It taught me that–it’s things that appear random, randomness is a subjective thing. Something can look random and unpredictable with one set of information; but if you have different information–like, if you understand the forces acting on the roulette ball and you can measure the position and velocity at a given point in time, then that thing that was previously random is no longer random.
And, that’s a lesson that I try to carry through the book. Because, what might not be predictable now–and economics could become predictable if we just have better models to make those predictions. If we use richer data, if we do it in a different way, then we can really change what’s possible in economics.
Russ Roberts: So, for people who may not know much about roulette, this key point is that you can still bet while the ball is moving, but then there’s a window there that closes, and that’s the window in which you got information–very imperfect–about where the ball might land. Even though it was imperfect, it gave you an edge over the House [the gambling company/hotel, which is allowed by law to take in a certain base percentage of profit–Econlib Ed.] that allowed you to be profitable.
As an aside, what’s interesting is that, of course, this was an extraordinary amount of investment. Intellectually–deeply satisfying. It’s a very–the book I mentioned about this episode is very entertaining. As is your account in this book. But, you should have become the wealthiest people in the history of the world because you had an edge over the House. Why didn’t that happen?
J. Doyne Farmer: Well, two reasons basically. Or maybe three reasons.
We were graduate students: we had a small bankroll. We actually started playing dimes and worked our way up to quarters, dollars, and so on. Which took a while.
Secondly, we had a lot of hardware failures. We were really pushing the envelope. And, this was contemporaneous with the very first Apple computers, but we were making ours a factor of a hundredths smaller or maybe factor of 20 smaller.
A third reason is that we were afraid of having our kneecaps broken. Back in those days, casinos were often owned by the mafia. There were well-documented stories of people being beaten up in the back room of the casinos.
And, the fourth was at some point we got interested in other things like chaotic dynamics, and it just became too irresistible to go back to graduate school and do that. We got our chance when we went–later on, there’s a sequel to The Eudaemonic Pie called The Predictors about beating the stock market, which we did successfully. Stock market has the advantage that they don’t throw you out of the casino for winning, and you don’t have to worry about getting your kneecaps broken. And, we did pretty well there.
Russ Roberts: It’s an interesting thing because if you ask–a colleague of mine asked me the other day–he said, ‘If I rolled dice and I rolled a six every time, a hundred times in a row, would that be a random outcome?’ And, some statisticians would say, ‘Well, it’s unlikely, but it still could happen.’ He was more interested in the fact that it was a pattern. But, let’s just say, are the dice fair? And, most people would say, ‘Well, obviously if you get a six 100 times in a row the dice aren’t fair.’ But, a statistician would have to say, in all honesty, ‘Well, it’s possible. It’s unlikely. It’s remote.’
But, owners of casinos have a different perspective on this. And, if you beat them night in and night out, they assume you’re cheating. Or that’s what they call cheating, actually. They don’t really care whether it’s random or not. They don’t want you in their casino anymore, right?
J. Doyne Farmer: Yeah. That’s right. Technically we weren’t cheating because we weren’t peeking at the cards or something like that.
Russ Roberts: Using a magnet to bring the ball under the–
J. Doyne Farmer: Everything we did at the time was legal. It’s actually not legal now. The reason is because Nevada passed a law against using a computer to predict the outcome of a game. And, unfortunately, I have to say that law was passed in part because of us.
Russ Roberts: Congratulations.
J. Doyne Farmer: Well, I don’t know if that’s a congratulations. Too bad for all the other people that could have done other similar systems.
Russ Roberts: There’s a chapter in the book about–there’s actually more than one–on climate change. But, you precede that chapter–you start that discussion off with a discussion of weather forecasting. I found that very, very interesting. Talk about the history of weather forecasting. It’s shockingly new. And, until you’ve actually read about it, as I did in your book, I didn’t really appreciate the challenge of it. Almanacs predicted weather, you know, forever. And they basically said, ‘Well, on March 6th, temperature has averaged this, so next March 6th, that’s probably going to be similar to that.’ And I thought, well, that’s what weather forecasting is, isn’t it? But, it’s worked–in the early days. How did it start? When did it start, and how has it changed, and why?
J. Doyne Farmer: Yeah. Well, people have been predicting weather for a long time. All the way back to Mesopotamia already we’re talking about how to predict the weather. But, we began to systematically predict the weather in the late 19th century. But the method was essentially a statistical method with some human intuition thrown in. And so, it was looking for analogies of similar weather patterns in the past and combining a few different things and then licking the finger and making a forecast. So, for roughly a hundred years, from the late 19th century to about 1980, the accuracy of weather forecasting stayed about the same. It maybe got a little better, but not much, because there was just an inherent limit to how well you can predict the weather using that kind of method.
Predictions of the weather got better starting in 1980 because they used a fundamental model. They actually modeled the physics of the weather. They put that on a computer and simulated the equations that describe the weather. And, with some other more heuristic things thrown in about cloud formation and heat transfer between the sea and the air and things like that that can’t be done cleanly from fundamental physics.
But, to make that work, there was actually an effort that started in 1950, was all the way from 1950 to 1980 to make it work. It involved an investment of billions of dollars. But, once it started working in 1980, it really paid off. Weather forecasts today are far better than they were in 1980. And, that actually has huge commercial significance, too, because planes crash due to bad weather, wars get lost due to bad weather, etc.
So, it’s an example of how we as human beings have banded together to do this. Because, weather prediction is also an international thing. Even now, the Russian weather stations collaborate with U.S. weather stations to produce better weather forecasts.
So, it’s just a remarkable example of how we can collectively benefit from making better predictions.
And, I bring it all up because I think we should do something similar in economics. It’s also an example of predicting from the bottom up, because weather forecasting doesn’t work at some high level of global temperature. You really need localized observations. You need a simulation that works from the bottom up on those localized observations because weather is complicated.
And, I draw an analogy in the book between fluid turbulence–which is what makes the weather unpredictable–and financial turbulence–which is what makes markets unpredictable. And, I discuss the many ways in which those two things actually are remarkably similar.
Russ Roberts: Yeah. And, I don’t know how accurate this is, but you say in the book that about every decade we gain a day in accuracy. Meaning a three-day forecast used to be the best we could really count on. Now it’s up to six. Something like that, right?
J. Doyne Farmer: Yeah. Maybe to put it just a bit differently: we could now, when–if a decade passes, we can forecast the forecast for one day into the future, are as good as they were for that one day backwards previously. In other words, if we could forecast with a certain level of accuracy two days ahead, a decade ago, we can now forecast with that same accuracy three days ahead.
Russ Roberts: And, yet, my weather app does 10 days effortlessly.
J. Doyne Farmer: Yeah. If you watch it for a while, you see it’s typically not very reliable. But, one day is not bad. It depends a bit on where you live. U.K. weather forecasts are worse than, you know, New York forecasts.
Russ Roberts: So, let’s talk about that. As you point out, it took billions of dollars of investment to get better at predicting the whether. That’s great for farming. It’s great for planning of all kinds–vacations and so on.
So, it was a pretty good investment. And, maybe we should do the same thing with economics.
Russ Roberts: I guess the question is: Do we really think it’s possible to get dramatically better? And, the standard way of thinking about this, which I think is maybe slightly misleading, is that well, people think and clouds don’t. So, people are going to be harder to predict than clouds. I don’t know if that’s the right way to think about it.
It seems to me the issue–and Hayek I think talks about this in his Nobel Prize address, The Pretense of Knowledge. He basically says: Let’s say there’s a hundred variables that affect the performance of a football player or an athlete in a team sport. We don’t have those data. We don’t know the conversation that the athlete had with the spouse the night before. We don’t know–all kinds of details that we might want to know we don’t have. And, I think he would say also that of course, they interact in all kinds of complex ways, so we can’t really anticipate what they’re going to cause; and therefore there’s an inherent non-predictability of economic macroeconomic events in that paper.
Do you disagree with that? Do you think that if we made the investments you’re talking about, we could clear some kind of hurdle in the complexity that would allow us to make more accurate predictions of the future?
J. Doyne Farmer: Yes. I do. On one hand, I agree with him that there are always going to be things that we can’t measure and there’s always going to be limits to how well we can predict. I’m not arguing in the book that we’re going to predict the economy the way we predict celestial mechanics, or even the weather.
Though, the weather is more challenging, I think, for other reasons. The weather is really complicated. The economy is actually simpler. The weather is fundamentally chaotic. We know that. So, that’s placing a limit on how well we can predict. But, the economy, I think in many ways is easier. The amount of computing we need to do the economy is actually less.
But, I think the key point is that there can be salient features that a allow predictability even when we don’t know all the details that Hayek is worried about.
Our COVID model was a good example. What did our COVID model depend on? It actually doesn’t really depend on human behavior at all. The only assumptions we make in there are that a company can’t produce its good if it doesn’t have the inputs, if it doesn’t have the labor, or if it doesn’t have the demand. Now human things come in on the demand part. The other things are pretty much physical. You can’t make steel if you don’t have iron. You can’t make steel if you don’t have the guy to run the converter that makes the iron. That doesn’t require much behavioral knowledge.
The demand is a little trickier, but nonetheless, we know kind of how demand would behave. We did make a few little mistakes because of that. For example, we thought that healthcare sector was going to actually go up in its gross output. It went down because routine procedures were postponed. We didn’t anticipate that. So, we made a few little mistakes like that.
But, the rest of it was really about understanding the way stuff flows through the economy. Because, we were able to predict–and maybe some behavior comes in this, too–we knew we had data saying for occupation by occupation at the level of about 500 occupations, how close do people work to each other in this occupation? So, therefore, we could see who is going to be able to go to work in each occupation.
Then we could look at which occupations were used in each industry, because we had a map of that. And then, we could predict how hard each industry was going to predict it was going to be hit as a result of people not being able to go to work, which was one of the fundamental drivers.
So, we’re only putting in behavior in a very weak way, but that did allow us to make a pretty good prediction about the shock that was going to happen to the economy. And then, we could watch–because our model was dynamic every day, the model would go along and ask the question, industry by industry: Does this industry have the labor it needs? Does it have the inputs it needs? Is there demand for its product?
And then, we just iterate it through day-by-day. We could watch the inventories running out for each industry. We could see the supply shocks propagating downstream, and the demand shocks propagating upstream. We could see them colliding. And that’s why the model worked well.
So, in other words, we got a salient feature in the economy during the COVID pandemic that didn’t depend on whether the football player was having an argument with his spouse. So, all those details didn’t matter. It really boiled down to a few salient things. And, so I think that’s why there’s hope for making predictions in other places.
Now, if you look at something like inflation or interest rates, they’re more complicated. Part of what we did in the COVID model, we said: ‘We’ll, just assume interest rates are staying constant. We’ll assume inflation won’t happen for at least a year.’ We were right about that. It did happen later. We expressed worries in our paper that over the long run, stuff is going to build up that could really cause problems. And those are harder problems. But I think those two are solvable.
This relates to something in physics called universality, which is: It’s well known in physics that there’s many situations in, say, solid state physics that underlies transistors and things like that, where the details don’t matter. As long as you’re in what’s called the right universality class, as long as certain criteria are met, the behavior is more or less the same. It’s only when you get outside of that universality class that it changes.
And, part of what people do is try and map out these universality classes. And, there are examples from some of my colleagues like Jean-Philippe Boucher’s group where they’ve actually mapped out the universality classes in macroeconomic models and shown that as long as things are kind of like this, this is what happens. But, if you cross a boundary, then something else happens. That really makes things a lot simpler because it means you don’t have to have all the details of everything just right. Just have to get certain things right.
Russ Roberts: Let’s close with a little more on the anthropology of what you’re trying to do compared to economists. You mentioned Rob Axtell of George Mason University. It’s one of the few places where academics are doing the kind of work you’re doing. I knew Rob. I met him. I was at George Mason. And we should have talked a lot, and we didn’t. Part of the reason he was not so close to where my office was, but some of it I think is anthropological. It’s that the jargon is different. Again, even though I was sympathetic to what he was doing, it was hard to talk. I’m curious if you interact much with mainstream economists and whether you feel that the two sides have something to learn from each other. I think most economists would think that physicists have nothing to teach them, and I wonder if physicists think economists have nothing to teach them. So, we can close with those reflections.
J. Doyne Farmer: Yeah. No. I certainly pay attention to mainstream economics. I’ve worked hard to learn the jargon. I think you have to understand your competition and keep a close eye on it, and dialogue is beneficial. I have close friends like John Giannakopoulos and Andrew Lowe, who are part of the mainstream. I was tickled that Larry Summers actually endorsed my book. I was quite surprised, actually. I sent him a copy of the book just because I mentioned his name several times, and I wanted to make sure I wasn’t saying anything that was wrong or offensive. He responded by saying, ‘Thanks for doing that. Most people don’t give me that opportunity.’ But, to my utter surprise, actually read the book and provided substantive feedback.
So, there are a few economists out there who are really open-minded, and who, by the way, typically have some criticisms or disagreements with their colleagues.
Now, it’s frustrating for me that the mainstream is not very willing in general to pay much attention to what we’re doing. I’ve gotten used to that. I’m resigned to publishing in second-tier journals because first-tier journals don’t publish this kind of work even when it’s really good. I’m resigned to the fact that my graduate students aren’t going to get jobs at Harvard for a while. I think in a decade or two, they may become the hottest thing around, but they’re going to have to hang in there until that happens. And, I think the mainstream is suffering by not paying more attention to us. We’re certainly trying to pay attention to them.
Russ Roberts: My guest today has been Doyne Farmer. His book is Making Sense Chaos: A Better Economics for a Better World. Doyne, thanks for being part of EconTalk.
J. Doyne Farmer: It was a pleasure. Thanks for a very intelligent discussion.