Health
AI in healthcare is just getting started
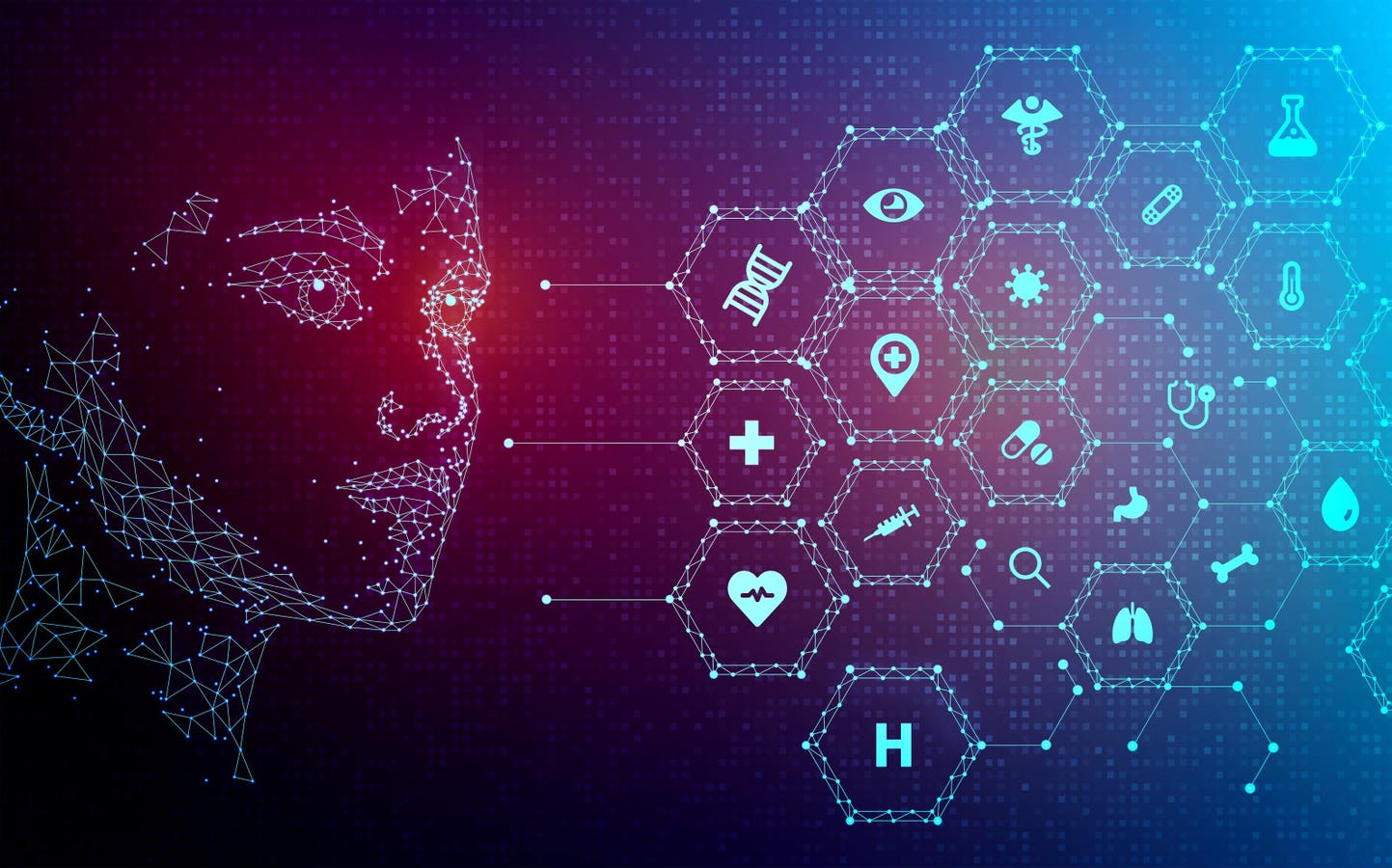
Artificial intelligence applications are becoming increasingly important in healthcare.
A new study published in Nature conducted a systematic analysis of the ethical landscape regarding the use and application of large language models in medicine and healthcare. The research shows that while LLMs have numerous advantages in data analytics, insight-driven decision making and information accessibility, issues of fairness, bias and misinformation are still of great importance in the healthcare context.
Artificial intelligence technology and the use of LLMs in healthcare have indeed grown exponentially, especially considering the speed at which the technology has developed over the past two years. While the launch of Chat GPT has catalyzed much of this work, the reality is that research into LLMs and the general integration of AI into industry use cases has been prevalent for decades.
Technology experts, privacy experts and industry leaders have raised concerns about the speed at which this work is progressing – growth that regulators simply have not been able to keep up with. So organizations and leaders alike are trying to develop frameworks to guide the development and ethical nuances for industry use cases. Take for example the Coalition for Health AI, also popularly known as CHAI, which aims to develop “guidelines and guardrails” to drive high-quality healthcare by promoting the adoption of credible, fair, and transparent healthcare AI systems. Another example is the Trustworthy & Responsible AI Network (TRAIN), led by Microsoft and European organizations to operationalize ethical AI principles and create a network where best practices related to the technology can be shared. The sheer amount of investment and resources being poured into initiatives like these indicate how important this agenda has become.
The reason for this emphasis is valid, especially in the context of healthcare use cases. AI in healthcare unlocks significant potential to simplify workflows, aid in insight-driven decision making, promote new methods of interoperability, and even make the use of resources and time more efficient. In the larger timeline, however, work on these applications is still relatively in its infancy. Furthermore, with respect to data reliability, LLMs are often considered only as effective as the datasets and algorithms with which they are trained. Therefore, innovators must continually ensure that the training data and methods used are of the highest quality. Furthermore, the data must be relevant, updated, bias-free, and supported by legitimate references so that systems can continue to learn as paradigms evolve and new data emerges. Even when there are pristine training conditions and all these criteria are met, AI systems can still often induce hallucinations, or generate content that is confidently claimed to be true but is often inaccurate. For an end user without a better source of truth, these hallucinations can prove to be harmful – and can become a major concern in the healthcare context.
Therefore, the increasing focus on ethical AI and the development of guidelines for AI are crucial aspects of cultivating this revolutionary technology, and will ultimately be paramount to truly realizing its potential and value in a safe and sustainable manner to unlock.